AI, Radiology and the Future

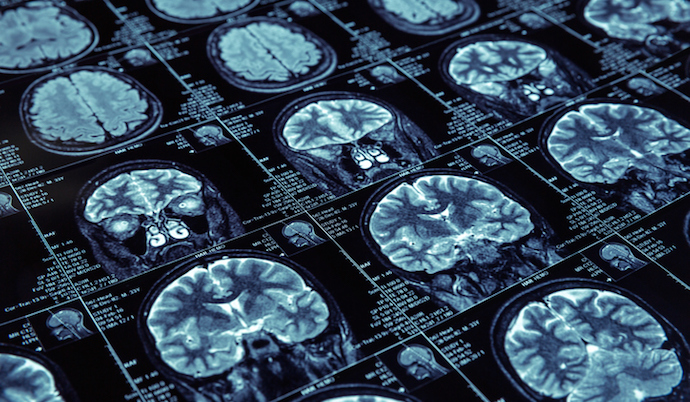
This article delves into how AI is revolutionizing Radiology and the potential future of healthcare. Learn how AI is streamlining diagnosis and revolutionizing the way medical professionals interact with patient data.
Introduction
With the advancement of Artificial Intelligence (AI) prevalent in recent years, it isn’t only IT spheres that can benefit from it. Healthcare is a massive industry, always looking for ways to progress and optimize their processes to ensure patients are treated quickly and correctly. By applying AI to healthcare, and by extension radiology, there are massive opportunities to revolutionize the industry in many ways, by using deep learning algorithms, convolutional neural networks and other data science techniques. In this article, the focus will be on radiology and what can be gained from AI, whilst identifying potential pitfalls.
AI’s Potential Impact on Radiology
So, what impact could AI have on Radiology itself? Other than broader benefits from its application in the everyday life of the humans behind the radiologist title, there are plenty of on-the-job applications that radiology AI can use to help appease existing issues and streamline work tasks.
Potential Applications
Not only does AI help with interpretation of data, but also can be transformative in other parts of the radiologist’s cycle, says Ryan K Lee, MD, MBA on Segmed’s Bytes of Innovation webinar. There’s a focus on making AI use a homogenous experience, with limited screen space for radiologists making it necessary to reduce their presence due to necessary applications such as PACS (Picture Archive and Communications System, a system that holds medical images) , dictation application and other medical imaging tools. This can be appeased by integrating the AI into the PACS itself, as a large majority of a radiologist’s time is spent in PACS and image analysis - up to 90% according to Lee.
This would most likely include a Machine Learning tool, one trained to pick up on discrepancies that the radiologist may have missed with their naked eye in the diagnosis process. Interventional radiology is also positively influenced by AI, helping to further solidify observations found by human or AI radiologists. Furthermore, AI algorithms can be deployed in student training, creating MRI images and other scans (by using machine learning algorithms) with abnormalities, and helping to teach students where to look and what to look for. Additionally, the dictation system can deploy AI, or widgets that are used by the radiologist, to further improve these systems.
Another area AI can greatly benefit the radiologists in their work is in ordering. AI tools can calculate the best scans to carry out based on what the patient is presenting with, and much faster than the human brain could. This reduces the time spent deciding on what scans to do, which benefits the whole process as well as increasing the accuracy of what scan is needed and reducing unnecessary scans, and showing what treatment is necessary. This can be implemented via a CDS (Clinical Decision Support) system.
Potentially, AI could also be used in scheduling as well - not only patients, but radiologists too. AI can match schedules with availability across many employees, and assign them to shifts based on these factors. It can see how many people are booked for appointments, and how historically busy that day and time of year is, and react accordingly.
Potential Problems and Issues
Deployment can cause problems as well, with various tools being deployed differently, and creating a problem with keeping track of these algorithms. There could be an issue with the back-end and support; vendors interacting with various elements of an IT setup or systems raises concerns over not only compliance with data standards, but the individual requirements set by each vendor, and how their policies intersect with the hospital’s own policies. The potential for a breach of clinical data is often not worth the risk due to the consequences involved with data breaches. Contracts pose an issue also, especially if they are provided by different companies for each algorithm, which may become confusing, causing problems when said contracts end by requiring reviews and increasing workload. Without a way to centralize the contract into one agreement, it can become a nightmare to deal with several different contracts that may intersect and clash with each other.
Resolutions and Necessary Adoptions
So what would be some standards that would increase the efficiency of AI in radiology whilst simplifying the processes used and required to set it up? Lee breaks AI implementation down into four sections.
Uniformity
Similar GUIs (Graphical User Interfaces) across multiple applications, which would allow radiologists to access AI software much easier whilst reinforcing that interface as the assistant tool no matter what application they are using.
Ease of Use
Making the AI easy to use and easy to learn decreases learning and adoption time and improves quality of life for the user.
Administration
Having a centralized platform where these applications and tools are housed creates an easier experience for users and potentially easier maintenance. Deployment is an essential piece of integrating new tools and systems.
Contracting
The use of a master contract can significantly decrease the review time necessary when it comes to renewing, whilst potentially reducing costs by buying a multi-tool deal rather than buying individual tools.
Conclusion
In conclusion, radiology and AI have a huge change on the horizon with the progression of technology pushing the limits of what can be done. Although it is early in the adoption process, there are positive signs that computer-aided diagnosis and other AI-related tools will massively benefit human radiologists and as it stands will not replace that human element in the radiology departments of hospitals worldwide.
AI technology is fast becoming an essential part of many people’s work and will continue to refine processes across healthcare as a whole. However, deployment has to be done right in order to maximise the talents of AI - and promote confidence and trust in this new technology, whilst improving patient care.
Footnotes
This is a summary of a webinar, titled 'Radiology, AI and the Future', which was a virtual event hosted online on Thursday October 6th, 2022. These webinars are a part of the Bytes of Innovation series, hosted by Segmed, which aims to host prominent and renowned researchers, physicians, and investors speaking about up-and-coming artificial intelligence in healthcare. You can find the talk at this link.